Monster galaxies' evolution from new HST mosaic image
After breaking its own record for the farthest star ever observed, the HST shows us its largest near-infrared image !
By using a fairly new technique with the HST known as Drift And SHift (DASH), the astronomers were able to capture multiple shots and combine them into a “mosaic”. FDASH also allowed them to take 8 pictures per Hubble orbit, effectively dividing by 8 the time required to cover the whole area that was imaged. This is a great improvement over the COSMOS-DASH image released in 2019 (0.66 deg2), since now the area is almost 6 times the apparent size of the Moon in our night sky (1.43 deg2) !
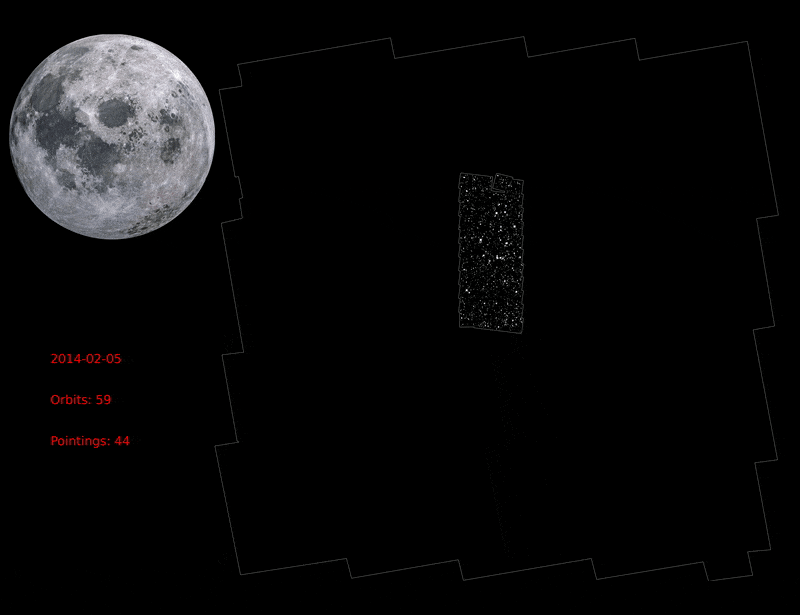
The researchers obtained this new 3D-DASH image with the HST's Wide Field Camera 3 (WFC3) in near-infrared wavelengths, which is useful to spot very distant galaxies, i.e., very early ones. In this huge set of galaxies, they seek to identify rare occurrences such as the Universe's most massive galaxies, highly active black holes and AGN, and galaxies on the verge of colliding and merging. One of the main authors of the study, who started working on the project in 2015 as a student, focuses on the first category of “monster” galaxies in an attempt to understand how these behemoths are formed and evolve.
Grid of some galaxy images from COSMOS-DASH Most massive star-forming galaxies in the 3D_DASJ survey
Another telescope that will observe in infrared wavelengths is the James Webb Space Telescope, however it was designed for close-up observations rather than to conduct such a wide survey. The next infrared space telescope with the capability to provide sharp wide-field images will be the Nancy Grace Roman Space Telescope, that should launch in the mid-2020s. For now, you, too, can explore an interactive online version of the 3D-DASH image in which you can move around the mosaic, zoom in and out, and look at it in specific infrared wavelengths.
Equally important to understand the structure of our Universe is having a good picture of the intergalactic medium (IGM). An incredible step forward was taken in that direction this week, thanks to the Instituto de Astrofísica de Canarias (IAC)’s new machine learning algorithm, Hydro-BAM. With it, they model Lyman-alpha forests: as light from a distant galaxy like a quasar journeys towards us, some of it gets absorbed by clouds (or, “trees”) of hydrogen gas, resulting in this Lyman-α set of lines in the quasar’s spectrum.
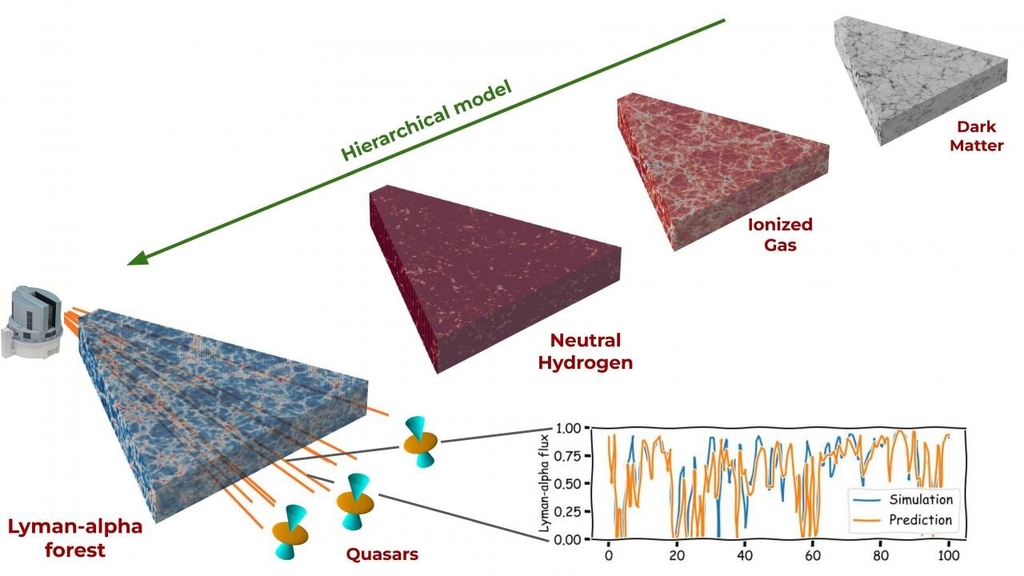
The upshot of the IAC’s research is not only that Hydro-BAM drastically reduces the computing time required for these simulations, but there is also physical insight. Namely, that the interactions of the ingredients of the IGM (hydrogen gas, dark matter and neutral hydrogen) affect the structure of our Universe on its largest scales, and they specifically do so in a hierarchical manner as illustrated above. We can then trace the evolution of structures and compare the results from the models to what we see today in observational surveys such as those conducted with the Dark Energy Spectroscopic Instrument or the Subaru Prime Focus Spectrograph.
Distant galaxies and the space between them still have a lot to reveal to us. Many more hours of observations, with a number of telescopes, are needed for us to complete our picture of the Universe's structures and their evolution.
Cover Image: Some galaxies in the 3D-DASH image, L. Mowla
Image Credits:
1 - DASH technique, Mowla et al. (2022)
2 - Some galaxies in the COSMOS-DASH image, Mowla et al. (2019) / Cutler et al. (2021)
3 - Galaxies in the 3D-DASH image, Mowla et al. (2022)
4 - Hierarchical model, F. Sinigaglia